Linear regression in Python#
Goals of this lecture#
Implementing linear regression in
statsmodels
.Interpreting model summaries:
Interpreting coefficients for continuous data.
Interpreting coefficients for categorical data.
Libraries#
## Imports
import pandas as pd
import numpy as np
import matplotlib.pyplot as plt
import statsmodels.api as sm
import statsmodels.formula.api as smf
import seaborn as sns
import scipy.stats as ss
%matplotlib inline
%config InlineBackend.figure_format = 'retina' # makes figs nicer!
Regression in Python#
There are many packages for implementing linear regression in Python, but we’ll be focusing on statsmodels
.
Loading our first dataset#
df_income = pd.read_csv("data/models/income.csv")
df_income.head(3)
Education | Seniority | Income | |
---|---|---|---|
0 | 21.586207 | 113.103448 | 99.917173 |
1 | 18.275862 | 119.310345 | 92.579135 |
2 | 12.068966 | 100.689655 | 34.678727 |
Exploratory visualization#
Does Education
correlate with Income
?
sns.scatterplot(data = df_income, x = "Education", y = "Income")
<Axes: xlabel='Education', ylabel='Income'>
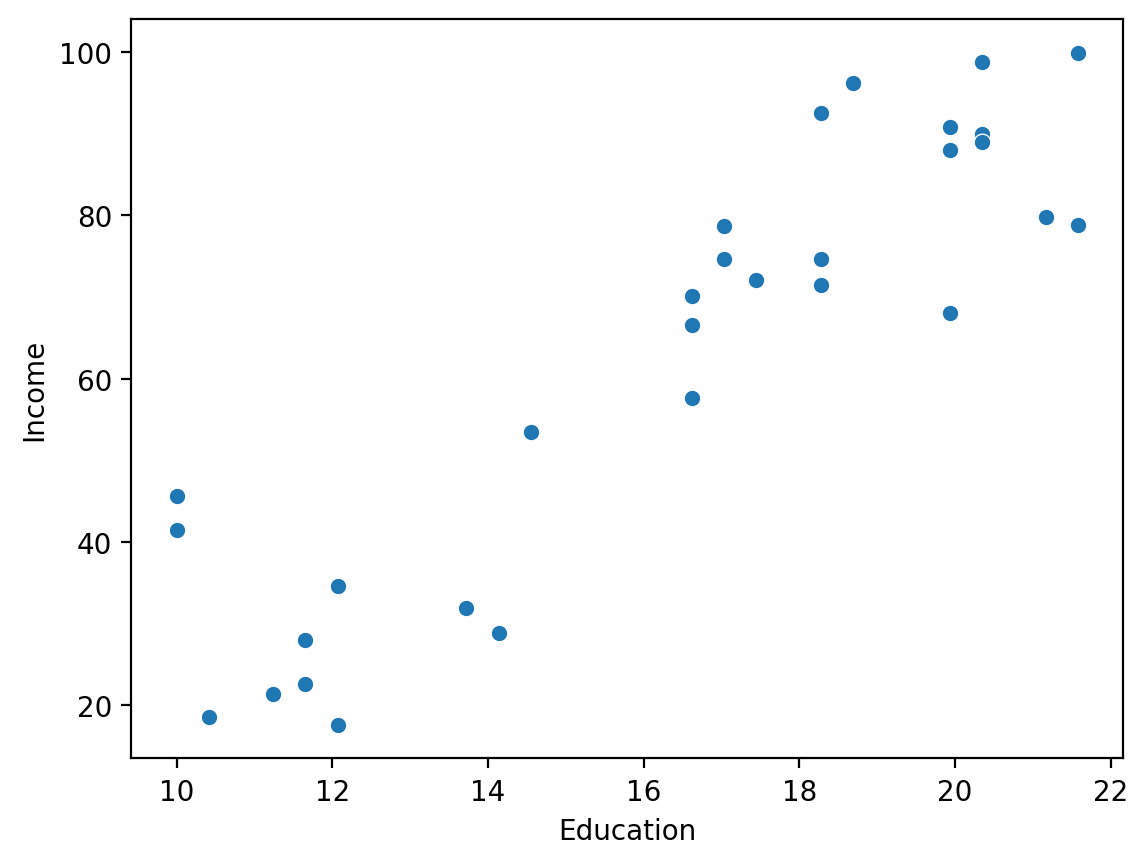
Building a regression model#
We can build a regression model using statsmodels.formula.api.ols
, here imported as smf
.
smf.ols(data = df_name, formula = "Y ~ X").fit()
mod = smf.ols(data = df_income, formula = "Income ~ Education").fit()
type(mod)
statsmodels.regression.linear_model.RegressionResultsWrapper
Inspecting output#
mod.summary() ### Lots of stuff here!
Dep. Variable: | Income | R-squared: | 0.812 |
---|---|---|---|
Model: | OLS | Adj. R-squared: | 0.805 |
Method: | Least Squares | F-statistic: | 120.8 |
Date: | Fri, 12 Jan 2024 | Prob (F-statistic): | 1.15e-11 |
Time: | 10:40:11 | Log-Likelihood: | -115.90 |
No. Observations: | 30 | AIC: | 235.8 |
Df Residuals: | 28 | BIC: | 238.6 |
Df Model: | 1 | ||
Covariance Type: | nonrobust |
coef | std err | t | P>|t| | [0.025 | 0.975] | |
---|---|---|---|---|---|---|
Intercept | -41.9166 | 9.769 | -4.291 | 0.000 | -61.927 | -21.906 |
Education | 6.3872 | 0.581 | 10.990 | 0.000 | 5.197 | 7.578 |
Omnibus: | 0.561 | Durbin-Watson: | 2.194 |
---|---|---|---|
Prob(Omnibus): | 0.756 | Jarque-Bera (JB): | 0.652 |
Skew: | 0.140 | Prob(JB): | 0.722 |
Kurtosis: | 2.335 | Cond. No. | 75.7 |
Notes:
[1] Standard Errors assume that the covariance matrix of the errors is correctly specified.
Extracing specific information#
## coefficients
mod.params
Intercept -41.916612
Education 6.387161
dtype: float64
## standard errors
mod.bse
Intercept 9.768949
Education 0.581172
dtype: float64
## p-values for coefficients
mod.pvalues
Intercept 1.918257e-04
Education 1.150567e-11
dtype: float64
Interpreting our model#
The linear equation#
Recall that the linear equation is written:
\(Y = \beta_0 + \beta_1 * X_1 + \epsilon\)
How would these terms map onto the coefficients below?
## Coefficients
mod.params
Intercept -41.916612
Education 6.387161
dtype: float64
Rewriting the linear equation#
We can insert our coefficients into the equation.
\(Y = -41.92 + 6.39 * X_1 + \epsilon\)
Which can then be used to generate a prediction for a given value of \(X\).
x = 20
y = -41.92 + 6.39 * x
print(y) ### our predicted value of Y, given X!
85.88
Check-in#
Write a function called predict_y(x)
which takes in a value x
and outputs a prediction based on these learned coefficients.
### Your code here
Solution#
def predict_y(x):
return -41.92 + 6.39 * x
predict_y(10)
21.979999999999997
predict_y(30)
149.77999999999997
Understanding the intercept#
The intercept term (\(\beta_0\)) is the predicted value of \(\hat{Y}\) when \(X = 0\).
What does this Intercept
value mean here?
## Coefficients
mod.params
Intercept -41.916612
Education 6.387161
dtype: float64
The intercept and the linear equation#
If \(X = 0\), the linear equation reduces to:
\(Y = -41.92 + \epsilon\)
predict_y(0) ### predicted value when x = 0
-41.92
Understanding the slope#
For a continuous variable, the slope is the predicted change in \(Y\) for every 1-unit change in \(X\).
What does this slope term mean here?
## Coefficients
mod.params
Intercept -41.916612
Education 6.387161
dtype: float64
Check-in: more practice with statsmodels
#
Build a regression model predicting Income
from Seniority
. What are the params
? What do they mean?
### Your code here
Solution#
mod_seniority = smf.ols(data = df_income, formula = "Income ~ Seniority").fit()
mod_seniority.params
Intercept 39.158326
Seniority 0.251288
dtype: float64
Check-in#
Why were these parameters chosen as opposed to some other \(\beta_0\) and \(\beta_1\)?
### Your response here
Solution#
Linear regression finds the set of parameters \(\beta\) that minimizes the residual sum of squares (RSS).
I.e., it finds the line of best fit!
Other relevant output#
statsmodels
also gives us:
A standard error associated with each coefficient.
A t-value associated with each coefficient.
A p-value associated with each coefficient.
A confidence interval associated with each coefficient.
Standard error#
These standard errors can be used to construct a confidence interval around our parameter estimate.
Assumption: parameters are estimates, which have some amount of normally-distributed error.
mod.bse ## Standard error associated with each coefficient
Intercept 9.768949
Education 0.581172
dtype: float64
Can report standard error as follows:
Education
was positively related withIncome
, \([\beta = 6.34, SE = 0.58]\).
t-value and p-value#
The standard error can also be used to calculate \(t\)-statistics for each coefficient, which can in turn be used to estimate a \(p\)-value to test for significance.
## t-values = params / bse
mod.params / mod.bse
Intercept -4.290801
Education 10.990148
dtype: float64
## double-checking our work
mod.tvalues
Intercept -4.290801
Education 10.990148
dtype: float64
## Check for significance
mod.pvalues
Intercept 1.918257e-04
Education 1.150567e-11
dtype: float64
Check-in#
Knowing what you know about standard error of the mean, how do you think our sample size affects the standard error for our coefficients?
### Your response here
Solution#
A larger sample (\(n\)) results in a lower standard error.
Coming up soon: We’ll discuss how to actually calculate standard error for our coefficients.
Confidence interval#
Finally, the conf_int
function return
s a confidence interval for all of our parameters.
This is calculated using the standard error.
By default, this assumes \(\alpha = .05\), i.e., a \(95\%\) CI.
Crucial assumption is that distribution of sample statistics (\(\beta\)) is normal.
## alpha = .05
mod.conf_int()
0 | 1 | |
---|---|---|
Intercept | -61.927397 | -21.905827 |
Education | 5.196685 | 7.577637 |
## alpha = .01
mod.conf_int(alpha = .01)
0 | 1 | |
---|---|---|
Intercept | -68.910782 | -14.922442 |
Education | 4.781232 | 7.993091 |
Check-in#
What is the \(95\%\) confidence interval for our coefficients for the model using Seniority
to predict Income
?
### Your code here
Solution#
These could be reported as follows:
Seniority
was positively related toIncome
, \([\beta = 0.25]\), \(95\%\) \(CI = [0.09, 0.41]\).
mod_seniority.conf_int()
0 | 1 | |
---|---|---|
Intercept | 21.714212 | 56.60244 |
Seniority | 0.090776 | 0.41180 |
Regression with categorical predictors#
What are categorical variables?#
A categorical (or qualitative) variable takes on one of several discrete values.
Examples:
spam
ornot spam
.male
orfemale
.right-handed
vs.left-handed
.smoker
vs.non-smoker
.treatment
vs.placebo
.Condition A
vs.Condition B
.
Note: some variables (e.g., handnedness
or gender
) are treated either categorically or continuously.
Example dataset: Stroop task#
In psychology, the Stroop effect is the delay in reaction time between congruent and incongruent stimuli.
df_stroop = pd.read_csv("data/models/stroop.csv")
df_stroop.head(3)
Condition | RT | |
---|---|---|
0 | Congruent | 12.079 |
1 | Congruent | 16.791 |
2 | Congruent | 9.564 |
Building a linear model#
How do we interpret these parameters?
mod_stroop = smf.ols(data = df_stroop, formula = "RT ~ Condition").fit()
mod_stroop.params
Intercept 14.051125
Condition[T.Incongruent] 7.964792
dtype: float64
Interpreting the slope for categorical variables#
\(\Large Y = \beta_0 + \beta_1X_1 + \epsilon\)
\(\beta_0 = 14.05\)
\(\beta_1 = 7.96\)
What is \(X_1\) here?
mod_stroop.params
Intercept 14.051125
Condition[T.Incongruent] 7.964792
dtype: float64
Using indicator variables#
A indicator variable (alternatively: “dummy variable”) represents the possible values of a categorical variable with different numbers, e.g.,
0
vs.1
for a binary variable.
In our case, this variable might take the form:
if
Condition == Congruent
–> 0if
Condition == Incongruent
–> 1
This is also called dummy coding or treatment coding.
Interpreting our equation with indicator variables (pt. 1)#
Our equation might thus look like this:
\(Y = 14.05 + 7.96X_{incongruent=1} + \epsilon\)
What happens when \(X\) is
Congruent
?What happens when \(X\) is
Incongruent
?
When Condition == Congruent
#
When Condition == Congruent
, the equation reduces to the Intercept
.
\(Y = 14.05 + 7.96 *0 + \epsilon = 14.05 + \epsilon\)
Note: This is actually identical to the mean
of the Congruent
condition!
df_stroop.groupby("Condition").mean()
RT | |
---|---|
Condition | |
Congruent | 14.051125 |
Incongruent | 22.015917 |
When Condition == Incongruent
#
When Condition == Incongruent
, the equation is the Intercept
plus the slope
.
\(Y = 14.05 + 7.96 * 1 + \epsilon = 14.05 + 7.96 + \epsilon\)
Note: The resulting value is the mean
of the Incongruent
condition.
14.05 + 7.96
22.01
df_stroop.groupby("Condition").mean()
RT | |
---|---|
Condition | |
Congruent | 14.051125 |
Incongruent | 22.015917 |
Check-in#
What does our slope
parameter reflect then?
Solution#
In univariate regression with a categorical, binary predictor, the slope reflects the difference in means between the two levels of that predictor.
sns.pointplot(data = df_stroop, x = "Condition", y = "RT")
<Axes: xlabel='Condition', ylabel='RT'>
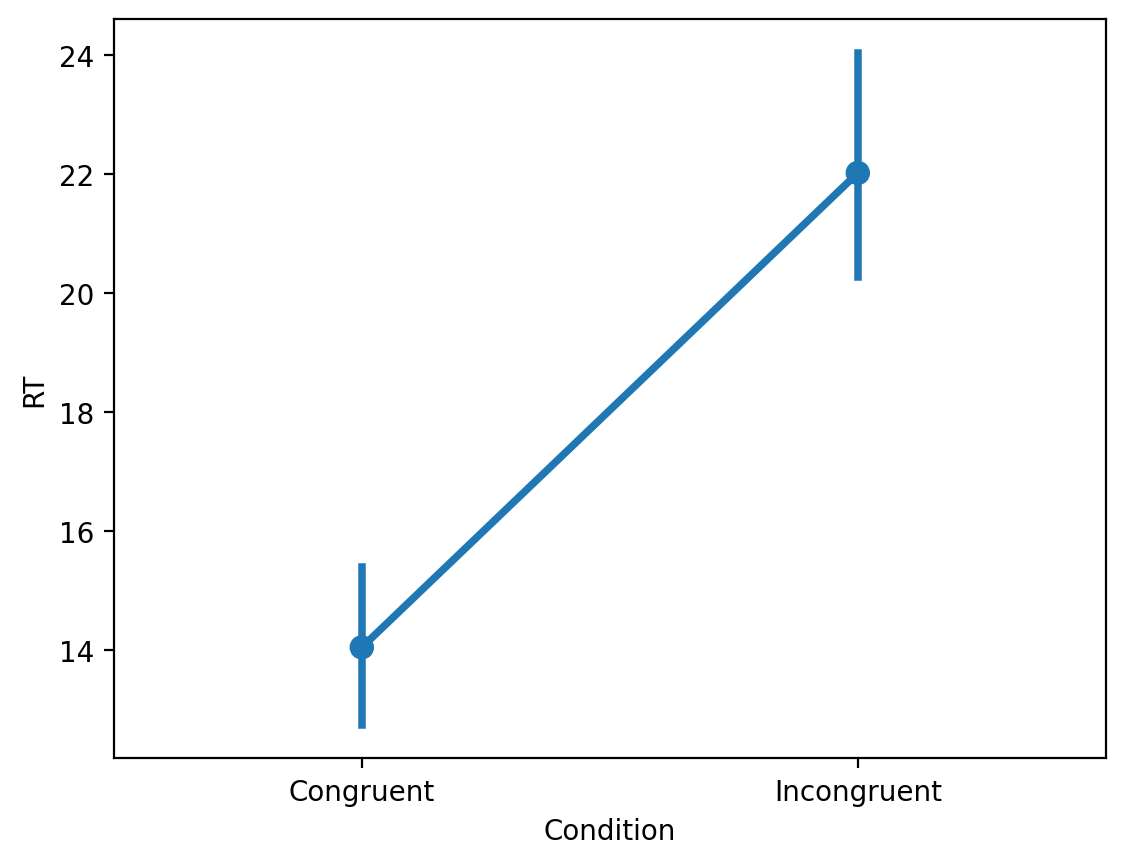
Check-in#
Load the tips
dataset from seaborn
and fit a linear model predicting tip
from time
.
Inspecting the model summary.
How should you interpret the resulting coefficients?
df_tips = sns.load_dataset("tips")
### Your code here
Solution#
The intercept reflects the mean tip
for Lunch
, and the slope reflects the difference in tip
amount between Lunch
and Dinner
.
mod_tips = smf.ols(data = df_tips, formula = "tip ~ time").fit()
mod_tips.params
Intercept 2.728088
time[T.Dinner] 0.374582
dtype: float64
df_tips[['time', 'tip']].groupby("time").mean()
tip | |
---|---|
time | |
Lunch | 2.728088 |
Dinner | 3.102670 |
Variables with \(>2\) levels?#
Many categorical variables have \(>2\) levels:
Assistant Professor
vs.Associate Professor
vs. `Full Professor.Conditions
A
,B
, andC
.Europe
vs.Asia
vs.Africa
vs.Americas
vs.Oceania
.
By default, statsmodels
will use dummy coding, choosing the alphabetically first level as the reference level.
Check-in#
Read in the dataset at
data/housing.csv
.Then, build a linear model predicting
median_house_value
fromocean_proximity
.Inspect the model
summary()
. How should you interpret the results?
### Your code here
Solution#
df_housing = pd.read_csv("data/housing.csv")
mod_housing = smf.ols(data = df_housing, formula = "median_house_value ~ ocean_proximity").fit()
mod_housing.params
Intercept 240084.285464
ocean_proximity[T.INLAND] -115278.893463
ocean_proximity[T.ISLAND] 140355.714536
ocean_proximity[T.NEAR BAY] 19128.026326
ocean_proximity[T.NEAR OCEAN] 9349.691963
dtype: float64
df_housing[['ocean_proximity', 'median_house_value']].groupby("ocean_proximity").mean()
median_house_value | |
---|---|
ocean_proximity | |
<1H OCEAN | 240084.285464 |
INLAND | 124805.392001 |
ISLAND | 380440.000000 |
NEAR BAY | 259212.311790 |
NEAR OCEAN | 249433.977427 |
How to code your variables?#
Coding refers to the approach taken to representing the different levels of a categorical variable in a regression model.
There are a variety of different approaches to contrast coding, some of which are summarized below.
Approach |
Description |
What is the |
What is the |
---|---|---|---|
Dummy coding |
Choose one level as reference. |
|
Difference between that level and |
Deviation coding |
Compare each level to grand mean. |
|
Difference between that level and grand mean. |
And many more!
By default, statsmodels
uses dummy coding.
Conclusion#
The
statsmodels
package can be used to build linear regression models.These models produce many useful summary features, including:
The fit \(\beta\) parameters (intercept and slope).
The standard error and p-value of those parameters.
Each \(\beta\) parameter has a particular interpretation with respect to \(\hat{Y}\).